Deep-Learning to Boost Biomedical Applications for Health
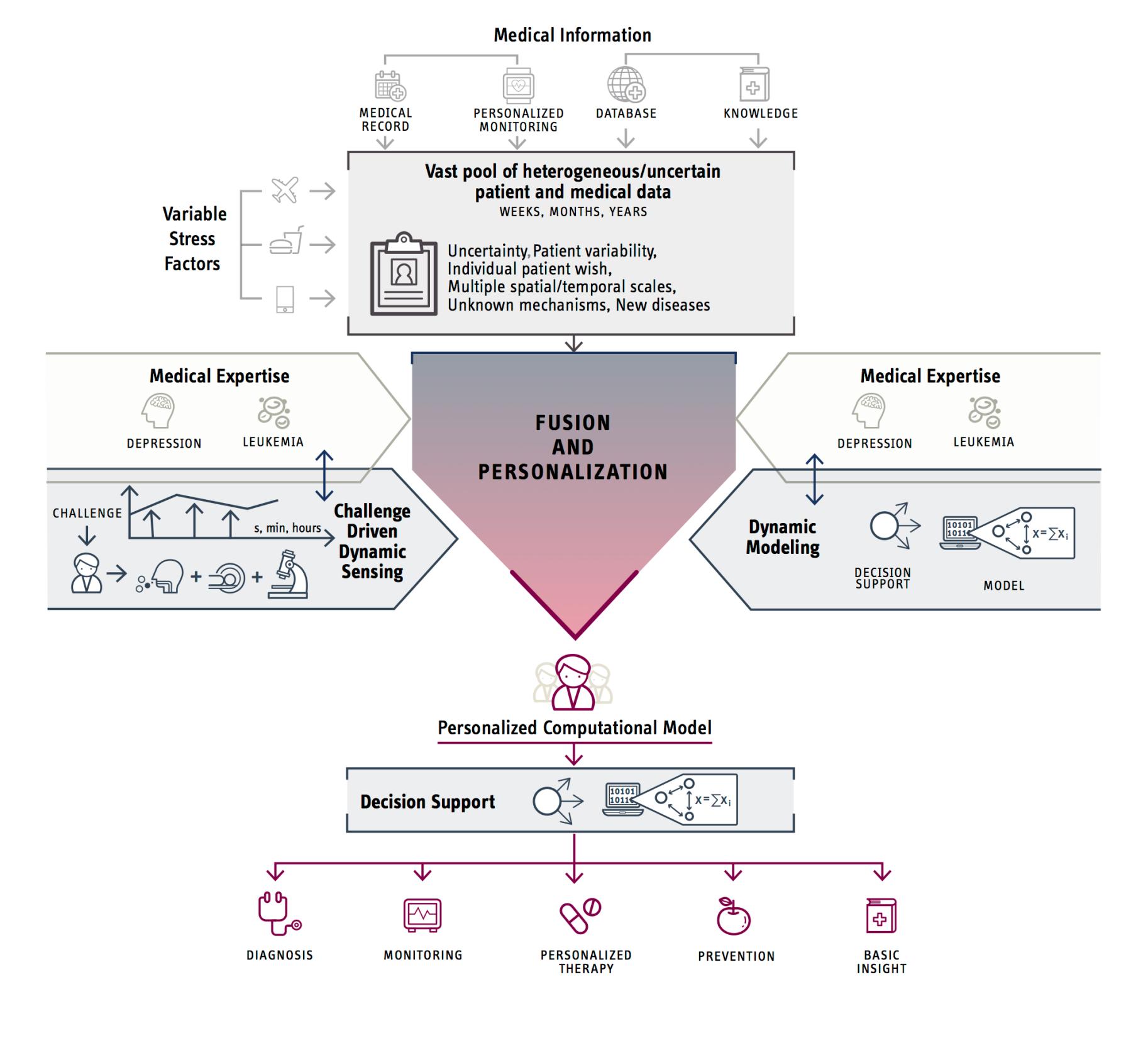
Depression is the most common psychiatric disorder and is associated with a tremendous burden of disease starting
already in early adulthood and in some cases even in childhood.
An ever-increasing amount of data (clinical, longitudinal, omics) from patients is available not only from treatment
in psychiatric services but also from treatment of other diseases in medicine. Moreover, monitoring of physical
activity and biological data during the disease course is possible through health monitoring systems. To reach
actionable knowledge, these data have to be analysed together and the longitudinal dynamics of these data have to
be considered in order to identify patterns that are relevant with respect to treatment of patients.
Within the framework of this project, we will set up dynamic predictive models for questions related to depression. The models
will fuse phenomenological modeling approaches with artificial intelligence approaches to allow for good outcome
prediction. The model and machine learning approaches will integrate the patients’ epidemiologic data, vital signs,
basic clinical parameters, laboratory parameters, and functional information, neuroimaging information and when
available omics information. Furthermore, based on the models we will provide decision support detecting the
different depression states and informing the medical doctor about possible treatment outcomes.
Area:
Machine learning, data science/analytics, systems medicine
Helpful/Required Prerequisites:
Core knwoledge of machine learning
Software: e. g. MATLAB, Python and/or R
Language: German and English.
Contact:
Nadine Rudolph
Anton Savchenko